Demystifying Machine Learning vs. AI vs. Deep Learning: A Comprehensive Comparison
Machine learning, artificial intelligence, and deep learning are terms that often appear in the media, but what do they actually mean? How are they related, and how do they differ from each other?
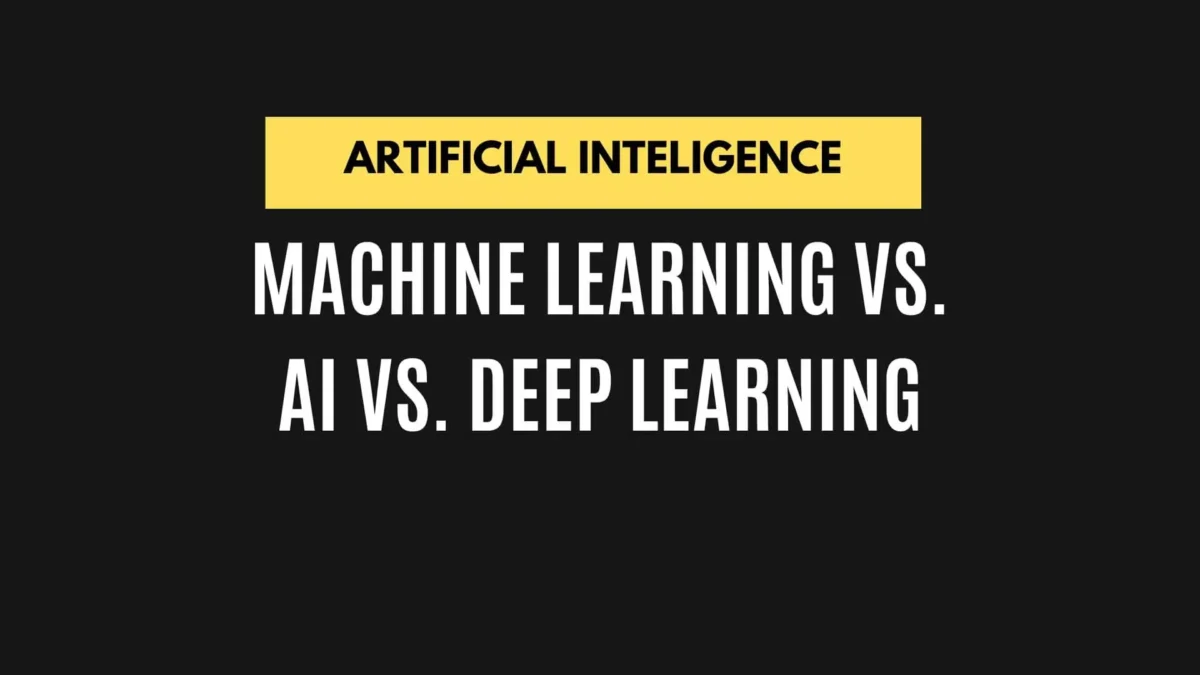
In this article, we’ll demystify these concepts and provide a comprehensive comparison of their definitions, applications, and challenges. We’ll also explore some of the most common examples of machine learning, artificial intelligence, and deep learning in action, and how they are transforming various industries and domains.
By the end of this article, you’ll have a clear understanding of the similarities and differences between machine learning, artificial intelligence, and deep learning, and why they matter for the future of technology and society.
Consider reading: Generative AI vs Predictive AI
Key Takeaways of Machine Learning vs. AI vs. Deep Learning
- AI encompasses various technologies that simulate human intelligence processes.
- Machine learning involves training algorithms to make predictions based on data.
- Deep learning uses neural networks with multiple layers for complex pattern recognition tasks.
- AI, machine learning, and deep learning have distinct functionalities and applications.
- Understanding the differences between AI, ML, and DL is crucial for leveraging the right technology.
- Artificial intelligence enables machines to mimic human cognitive functions and optimize complex tasks.
Machine Learning vs. AI vs. Deep Learning Examples:
The landscape of technology is rapidly evolving with advancements in Artificial Intelligence (AI), Machine Learning (ML), and Deep Learning (DL). These technologies, while interconnected, serve unique purposes across different sectors. Let’s demystify them by delving into practical examples that highlight their distinct applications and impact:
AI in Action:
- Chatbots Enhancing Customer Support: Imagine interacting with a virtual assistant that understands and responds to your queries in natural language. This is AI at work, streamlining customer service.
- Fraud Detection Systems: Financial institutions leverage AI to scrutinize transactions in real-time, pinpointing anomalies that could indicate fraud.
- Personalized Entertainment: Streaming services use AI to tailor recommendations, creating a personalized viewing experience by analyzing your preferences.
Machine Learning at Work:
- Combatting Spam: Email platforms employ ML algorithms to filter out spam, ensuring your inbox stays relevant and clutter-free.
- Predictive Maintenance in Manufacturing: By predicting equipment failures before they happen, ML minimizes downtime and maintenance costs.
- Credit Scoring for Loans: Financial entities apply ML to evaluate loan applications more accurately, basing decisions on complex data analyses.
Deep Learning’s Groundbreaking Applications:
- Autonomous Vehicles: DL powers the image recognition capabilities in self-driving cars, enabling them to identify pedestrians and signs, paving the way for safer roads.
- Transforming Speech Recognition: From voice-activated assistants to real-time transcription, DL revolutionizes how machines interpret human speech.
- Advancements in Healthcare: DL algorithms analyze medical imagery with unprecedented accuracy, aiding in the early detection and diagnosis of diseases.
Through these examples, we see how AI offers a foundation for simulating human intellect, ML specializes in learning from data to make informed predictions, and DL excels at deciphering intricate patterns through its sophisticated neural networks.
By showcasing the real-world impact of AI, ML, and DL, we aim to provide a clear understanding of their distinctive roles and potential, moving beyond the technical terms to appreciate their transformative power in various domains.
Consider reading: AI in Healthcare: Innovations and Future Trends
Machine Learning vs. AI vs. Deep Learning: Is There a Difference?
When comparing AI, machine learning (ML), and deep learning (DL), it’s crucial to understand the distinctions in their functionalities and applications.
Artificial Intelligence (AI):
- AI focuses on simulating human intelligence processes.
- It involves the development of systems capable of performing tasks that typically require human intelligence.
- Examples include chatbots, personalized recommendations, and fraud detection systems.
Machine Learning (ML):
- ML is a subset of AI that focuses on developing algorithms allowing computers to learn and make decisions based on data.
- It uses statistical techniques to enable machines to improve performance on a specific task over time.
- Applications include email filtering, predictive maintenance, and credit scoring algorithms.
Deep Learning (ML):
- DL is a specialized subset of ML that mimics the workings of the human brain in processing data and creating patterns for decision-making.
- It involves neural networks with multiple layers to extract high-level features from raw data.
- Examples include image recognition, speech recognition, and medical diagnosis tools.
Machine Learning vs. AI vs. Deep Learning Key Differences:
- AI encompasses the broader concept of creating systems capable of human-like tasks.
- ML is the subfield of AI focused on developing algorithms that improve automatically through experience.
- DL is a subset of ML that utilizes deep neural networks to analyze data and make decisions.
Understanding these distinctions is essential in leveraging the right technology for specific applications.
What is Artificial Intelligence?
Artificial intelligence, often referred to as AI, is a technology that enables machines to mimic human cognitive functions and intelligence processes. This includes tasks such as problem-solving, learning, and decision-making. AI leverages predictions and automation to optimize and solve complex tasks that were traditionally performed by humans.
- AI encompasses machines simulating human intelligence processes.
- It optimizes tasks like facial and speech recognition, decision-making, and translation.
- AI is categorized into Artificial Narrow Intelligence (ANI), Artificial General Intelligence (AGI), and Artificial Super Intelligence (ASI).
Understanding artificial intelligence is essential to grasp the broader world of technologies like machine learning and deep learning. Each of these technologies plays a unique role in the area of artificial intelligence.
What is Machine Learning?
Machine learning is a subset of artificial intelligence.
- In most cases, machine learning refers to traditional machine learning.
- Deep learning specifically refers to multilayered neural networks.
In machine learning:
- Engineers manually design and predefine features to represent the data numerically.
- These features are based on the engineer’s knowledge and understanding of the data.
- Features like the number of eyes, legs, and heads can represent animals.
- Handcrafted features represent the data in machine learning.
- Making predictions based on patterns and inferences without explicit programming.
- Utilizing statistics as a driving force behind the algorithms.
- Learning to make predictions through the analysis of data patterns.
What is Deep Learning?
Deep learning is a sophisticated subset of machine learning and artificial intelligence. It uses intricate neural networks with multiple layers to process data and make complex decisions. Here are key points to understand deep learning:
- Deep learning networks consist of many layers, making them more advanced than traditional neural networks.
- These networks are powered by components such as a robust power supply, a GPU, and a large RAM.
- Deep learning models require substantial training time due to their complexity, unlike neural networks that demand minimal training.
In the area of artificial intelligence, it’s crucial to grasp the role of deep learning as a powerful tool within the broader world of machine learning and AI.
Consider reading: Future of AI in Education
Final Thoughts on Machine Learning vs. AI vs. Deep Learning
Deep learning is a modern direction within the scope of artificial intelligence and machine learning. Modern neural networks used in this technology with their complex decision mechanisms are unlike classic approaches. These advanced deep learning models bring out the demand for high resources like GPU and a huge size of RAM.
Working in today’s dynamic world of AI, it becomes highly important for one to understand the importance of deep learning. The power of social data lies in the understanding of its role and potential for driving innovation and the general advancements in various industries.
Such being the case, it is this very embracing of deep learning as a pivotal tool in the AI ecosystem that will allow for the surfacing of new possibilities that ought to be embraced to push what may be otherwise limiting technology.
FAQs on Machine Learning vs. AI vs. Deep Learning
What is the difference between AI and machine learning and deep learning?
Artificial Intelligence (AI) is the overarching concept of machines simulating human intelligence. Machine Learning (ML) is a branch of AI focused on algorithms that enable machines to learn from data. Deep Learning, a subset of ML, employs neural networks with many layers to interpret large, complex datasets for more sophisticated insights.
What is AI vs ML vs DL vs DS?
AI, or Artificial Intelligence, encompasses technologies that mimic human intelligence. Machine Learning (ML) is a segment of AI focused on teaching machines to learn from data without explicit programming. Deep Learning (DL), a branch of ML, uses artificial neural networks to analyze complex data. Data Science (DS) integrates various fields, including AI, to derive meaningful insights from data.
Should I learn AI or ML?
Your choice between AI and ML depends on your interest areas. If you’re drawn to robotics or computer vision, AI is more suitable. However, for a specific career in data analysis and algorithms, machine learning (ML) might be the better path. Consider your career goals and select the field that aligns more closely with them.